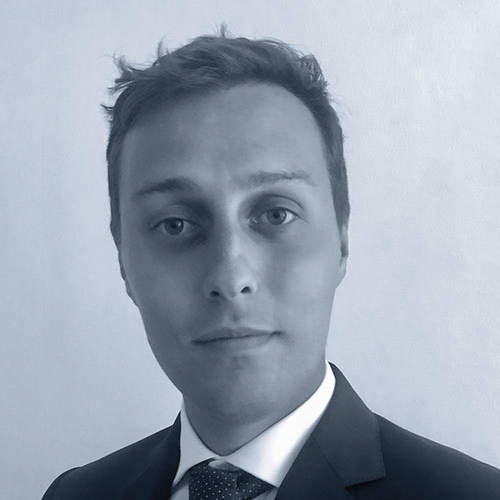
Ludovico Nista
RWTH Aachen University,
Institute for Combustion Technology
Contact
RWTH Aachen University
Institute for Combustion Technology
Templergraben 64
52056 Aachen
energy_conversion@nhr4ces.de
Biography
Ludovico is a research assistant at the Institute for Combustion Technology of the RWTH Aachen University. He has been a research fellow at Von Karman Institute for Fluid Dynamics and visiting scholar at Technion – Israel Institute of Technology, after receiving his Master of Science in Mathematical Engineering and obtaining a Master of Research in Fluid Mechanics at the Von Karman Institute for Fluid Dynamics.
His main research interests are in the fields of machine learning theory, turbulent combustion, and high-order numerical methods with application to applied energy systems.
Since 2020, Ludovico is a member of the SDL Energy Conversion for the National High Performance Computing Center for Computational Engineering Sciences (NHR4CES).
Thematic Advice
Predictive simulations of turbulent combustion are crucial to the design of energy-conversion systems in the transportation, power, and defense sectors, among others. Due to their multiscale, multiphysics nature, these systems are typically intractable to simulation at full resolution. Lower- resolution simulations are possible but require closure models; currently, state-of-the-art closure models fail to capture key dynamics in certain regimes of turbulent combustion, and the use of these models can lead to incorrect predictions.
The application of machine learning methods, already successfully employed in other research areas (e.g., image processing, speech recognition, or video classification), could provide insight to move beyond classical equation-based closure models. Ludovico is trying to develop some new frame- works and methodologies to improve machine learning capabilities by incorporating physics into the process, to uncover new physical understanding.
Professional Competence
Ludovico is seeking to develop a data-driven Super-Resolution (SR) model for the unresolved stress and scalar flux tensors in the context of Large Eddy Simulations, aiming to partially replace available equations-based models. The need for a reliable, accurate, and robust Machine Learning (ML) framework advocates for a strong effort to explore a-posteriori performance and feasibility of apply- ing SR methods, by achieving a certain degree of generalizability and numerical stability.
In this view, the combination of high-performance computing techniques with high-level ML archi- tectures is fundamental for exploiting model-consistent training to stabilize the simulation and to ensure generalization capabilities, remaining two of the major obstacles in broadening the applica- tions of such data-driven SR models.
He is also an active member of the numerical turbulent as well as combustion community and fre- quently participates as a speaker at the most prestigious conferences and meetings of the fields.