Our researchers from the CSGs and SDLs are very active at national and international conferences and present their research to the specialist communities. Heinz Pitsch and Ludovico Nista from our SDL Energy Conversion actively participated in the 1st workshop on machine learning for fluid dynamics in Paris.
Heinz Pitsch gave a plenty talk on “Advancing Turbulent Combustion Modeling for Future Renewable Fuels: Unveiling the Potential of Super-Resolution Generative Adversarial Networks.” Turbulent combustion is highly complex because of the multitude of independent time and length scales and the strong non-linearities associated with combustion chemistry. As a result, modeling based on physical insights and rigorous simplifications is difficult. The topic becomes even more challenging with future renewable fuels with entirely different combustion properties compared with fossil energy carriers. Machine-learning-based modeling approaches provide an opportunity for improving the accuracy and usability of large-eddy simulations of chemically reacting flows. The plenary talk discussed the application of data-driven super-resolution models based on generative adversarial networks, highlighting several interesting features. The potential usage of adversarial training, different training strategies, and their practical applications were also discussed, with a focus on enhancing out-of-sample reconstructions.
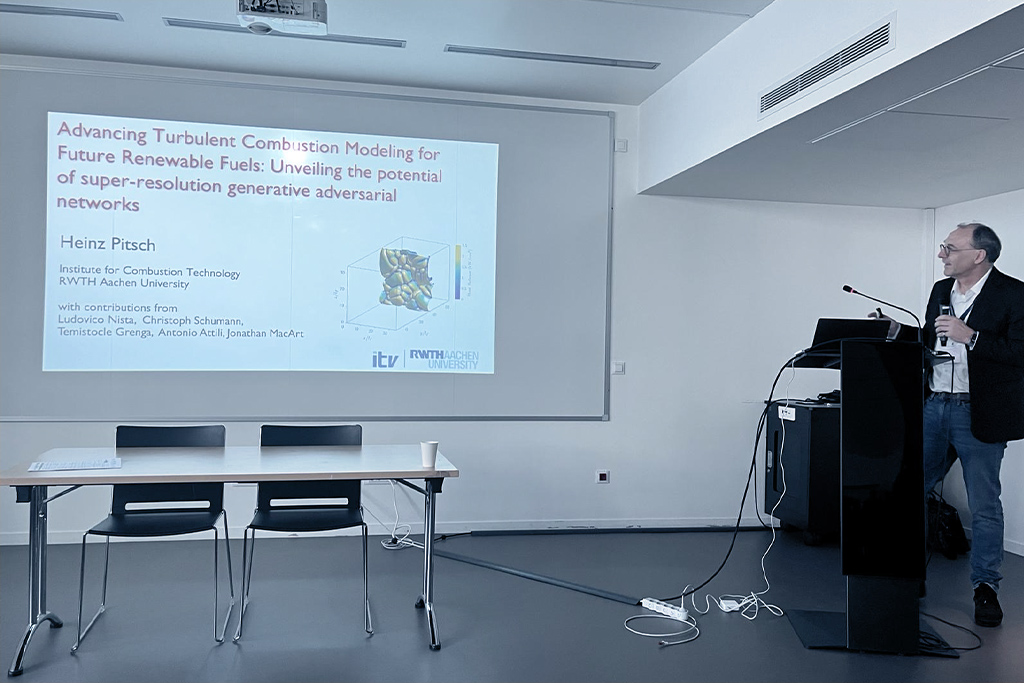
Heinz Pitsch’s plenary lecture titled “Advancing Turbulent Combustion Modeling for Future Renewable Fuels: Unveiling the Potential of Super-Resolution Generative Adversarial Networks.”
Ludovico’s talk titled “Influence of Adversarial Training On Super-Resolution Turbulence Reconstruction” highlighted the ongoing challenge of accurately reconstructing turbulent flow fields. Traditional supervised-CNN methods face difficulties in capturing high-frequency and small-scale features accurately, prompting a shift towards GANs due to their adaptability through adversarial training techniques. The research showcases the superiority of GAN-based architectures over supervised CNNs in handling in-sample scenarios, with the discriminator network playing a pivotal role in bolstering out-of-sample robustness. Particularly noteworthy is the improved reconstruction of small-scale gradients and turbulence intermittency, indicating that adversarial training represents a promising avenue for enhancing reconstruction and extrapolation capabilities within turbulent flow modeling.
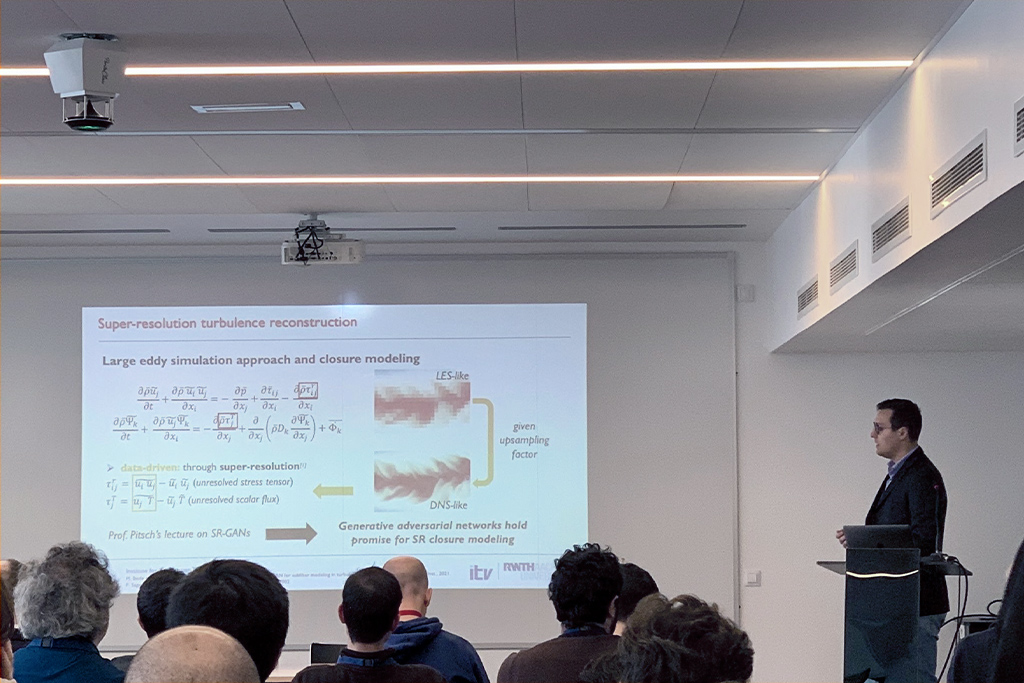