After last year’s Community Workshop provided a general introduction of our Cross-Sectional Groups (CSG) and Simulation and Data Labs (SDL), our second Community Workshop focused on the SDLs Fluids and Energy Conversion and the CSG Data Science and Machine Learning. This year’s topic was Machine learning in Computational Fluid Dynamics. About 65 people attended the online workshop on February 28 and March 1, 2023.
You can download the presentations below.
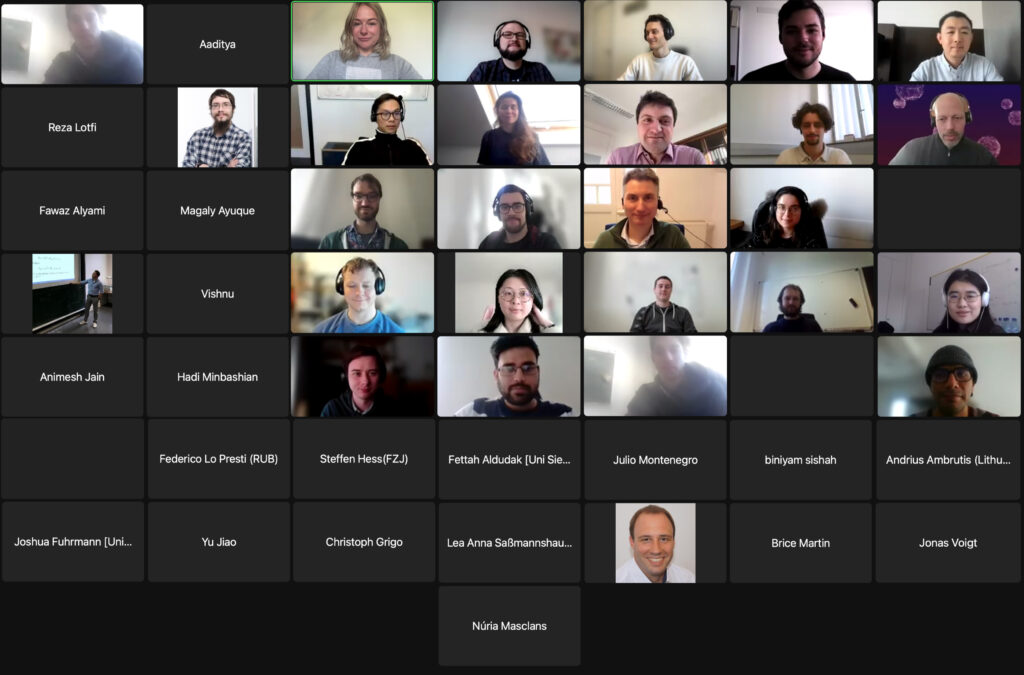
The first speaker, Dr. Andre Weiner, provided an introduction to the optimization of closed-loop flow control laws by means of deep reinforcement learning (DRL) in the context of computational fluid dynamics (CFD).
Afterwards, Dr. Mengwu Guo discussed several probabilistic options for the learning of reduced-order models, in which a significantly reduced dimensionality of dynamical systems guarantees improved efficiency, and the facilitated uncertainty quantification certifies computational credibility.
Mr. Jeremy T.P. Karpowski & Mr. Ludovico Nista started day two with their joined talk: Deep learning architectures for thermochemistry and subgrid closure modeling in turbulent combustion. The first part of the talk focused on the development of an artificial neural network chemistry manifold to simulate a premixed methane-air flame undergoing side-wall quenching. The second part focused on the development of a physics-informed super-resolution generative adversarial network (PIESRGAN) employed for the unresolved stress and scalar-flux tensors closure modeling.
During Dr. Corentin Lapeyre’s talk Leveraging AI for better high-fidelity CFD without compromising accuracy and reliability he showcased some applications of these new approaches and discussed some promising avenues for more efficient hybrid solvers in the near future.
As the last speaker, Prof. Andrea Beck gave an overview of recent successes (and some failures) of combining modern, high order discretization schemes of Discontinuous Galerkin (DG) type with machine learning submodels and their applications for large scale computations.
The workshop concluded with a panel discussion with the invited speakers, Prof. Beck, Dr. Weiner, Dr. Lapeyre, Mr. Nista, and Mr. Karpowski.
These experts in the fields of computational fluid dynamics (CFD), machine learning (ML), and numerical solvers, discussed several topics related to the integration of ML models into CFD simulations. The moderator, Prof. C. Hasse, opened the panel by introducing the panelists and the topics they would be discussing.
The first topic explored was the perspective and challenges of data-driven modeling coupled with standard equation-based models. The panelists agreed that while data-driven modeling offers valuable insights, it should not replace equation-based models entirely. Instead, the two approaches should be used together to complement each other’s strengths.
The second topic focused on efficiently embeding ML models into numerical solvers, with a specific emphasis on unbalanced heterogeneous high-performance computing (HPC) infrastructures. The panelists discussed various optimization techniques for ML models for HPC, such as parallelization. They also emphasized the importance of designing ML models that can handle unbalanced data, infrastructure, and various boundary conditions.
The panelists also delved into ensuring the robustness of a-posteriori ML architectures, specifically regarding generalizability. They emphasized the need to validate ML models on a variety of datasets, to be transparent about their limitations, and to develop metrics to evaluate ML models’ accuracy.
The final topic explored how to prepare future ML scientists in the field of CFD and address the black-box nature of ML applications. The panelists emphasized the importance of interdisciplinary collaboration between CFD and AI experts. They also highlighted the need for making ML models explainable and interpretable to ensure their reliability.
Overall, the panel provided valuable insights into the challenges and opportunities presented by the integrating ML models into CFD simulations, emphasizing the importance of collaboration and careful consideration of the strengths and limitations of each approach.
Below you find all presentations from the NHR4CES Community Workshop.