News from our Simulation and Data Lab Materials Design: Have a look at the article “A deep learned nanowire segmentation model using synthetic data augmentation“, which was recently published in Npj Computational Materials and in the interactive segmentation tool. The computing time for this research was provided on the supercomputer Lichtenberg II at Technische Universität Darmstadt Automated particle segmentation and feature analysis of experimental image data is a big challenge in data-driven material science. In the present work, the SDL Materials Design applies synthetic images that resemble the experimental images in terms of geometrical and visual features, to train the state-of-art Mask region-based convolutional neural networks (Mask R-CNN) to segment densely packed nanowires from battery electrode materials in different types of microscopy images. The proposed methodology can be extended to any optical intensity-based images of variable particle morphology, material class, and beyond… read more. The research was done by Binbin Lin and his collaborators.
15. May 2022
News from SDL: Have a look at the new article
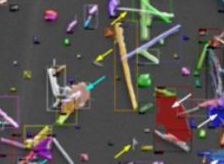